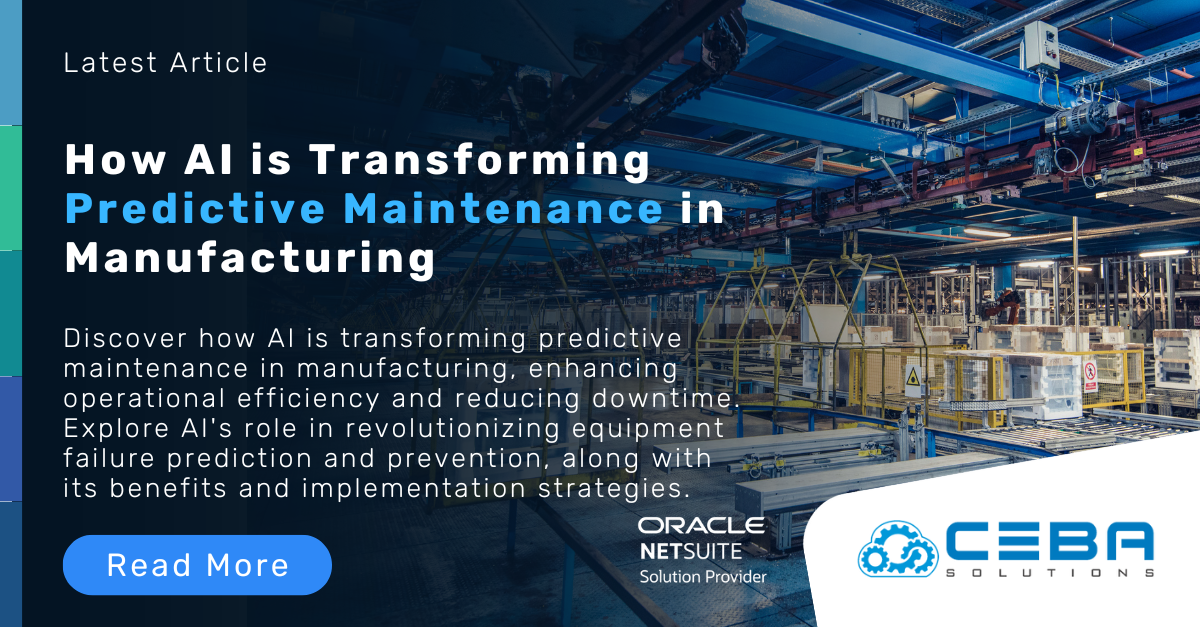
How AI is Transforming Predictive Maintenance in Manufacturing
How AI is Transforming Predictive Maintenance in Manufacturing
In the realm of manufacturing, ensuring the optimal performance and longevity of machinery is crucial for maintaining productivity and minimizing costs. Traditional maintenance strategies—reactive and preventive—have their limitations, often leading to unexpected equipment failures or unnecessary maintenance activities. Enter predictive maintenance, a game-changer powered by artificial intelligence (AI) that promises to revolutionize how manufacturers maintain their equipment. By leveraging AI, predictive maintenance transforms data into actionable insights, enabling timely interventions and significantly enhancing operational efficiency. This article delves into the transformative impact of AI on predictive maintenance in manufacturing, exploring its benefits, implementation strategies, and future potential.
Understanding Predictive Maintenance
Predictive maintenance (PdM) is a proactive approach that uses data analytics to predict when equipment failures might occur and schedule maintenance activities accordingly. Unlike reactive maintenance, which deals with repairs after a failure, or preventive maintenance, which follows a fixed schedule, PdM aims to perform maintenance only when necessary, based on the actual condition of the equipment.
The Role of AI in Predictive Maintenance
AI enhances predictive maintenance through advanced data analysis, machine learning algorithms, and real-time monitoring. Here’s how AI contributes to the effectiveness of PdM:
- Data Collection and Integration:AI-powered systems collect vast amounts of data from various sources, including sensors, IoT devices, and historical maintenance records. This data encompasses parameters like temperature, vibration, pressure, and operational logs, providing a comprehensive view of equipment health.
- Advanced Analytics and Machine Learning:Machine learning algorithms analyze the collected data to identify patterns and correlations that signify potential equipment failures. These algorithms can learn from historical data to improve their predictive accuracy over time. Techniques such as anomaly detection, regression analysis, and classification are employed to forecast maintenance needs accurately.
- Real-Time Monitoring and Alerts:AI enables continuous real-time monitoring of equipment. When the system detects deviations from normal operating conditions, it generates alerts, allowing maintenance teams to intervene before a minor issue escalates into a major failure. This proactive approach helps prevent unexpected downtime and costly repairs.
- Predictive Modeling:AI creates predictive models that estimate the remaining useful life (RUL) of equipment components. These models use data-driven insights to predict when a component is likely to fail, allowing maintenance to be scheduled at the most opportune time, thereby extending the lifespan of machinery and optimizing maintenance resources.
Benefits of AI-Driven Predictive Maintenance
The integration of AI into predictive maintenance offers several significant benefits for manufacturers:
- Reduced Downtime:By predicting and preventing equipment failures before they occur, AI-driven PdM minimizes unplanned downtime. This ensures that production schedules are maintained, and operational disruptions are kept to a minimum.
- Cost Savings:Predictive maintenance reduces the need for emergency repairs and minimizes the frequency of routine maintenance, leading to substantial cost savings. It also optimizes spare parts inventory by identifying which parts are likely to fail and when.
- Enhanced Equipment Lifespan:Timely maintenance interventions based on AI predictions help extend the operational life of equipment. This reduces the need for premature replacements and maximizes the return on investment for machinery.
- Improved Safety:AI-driven PdM enhances workplace safety by identifying potential equipment failures that could pose safety hazards. By addressing these issues proactively, manufacturers can create a safer working environment for their employees.
- Data-Driven Decision Making:AI provides maintenance teams with actionable insights and data-driven recommendations. This empowers decision-makers to prioritize maintenance activities based on actual equipment conditions, improving overall maintenance efficiency.
Implementing AI for Predictive Maintenance
Successfully implementing AI-driven predictive maintenance involves several key steps:
- Data Infrastructure:Establish a robust data infrastructure to collect, store, and process data from various sources. This includes installing sensors and IoT devices on critical equipment and integrating data from existing maintenance management systems.
- Algorithm Development:Develop and train machine learning algorithms using historical data. Collaborate with data scientists and AI experts to create models tailored to the specific needs of your equipment and operations.
- Real-Time Analytics:Deploy real-time analytics platforms that can process incoming data and generate alerts when anomalies are detected. Ensure that these platforms are integrated with your maintenance management system for seamless workflow management.
- Continuous Improvement:Continuously monitor the performance of AI models and update them with new data to improve their accuracy over time. Implement feedback loops where maintenance outcomes are used to refine predictive algorithms.
- Training and Change Management:Train maintenance staff to understand and use AI-driven tools effectively. Foster a culture of innovation and continuous improvement, encouraging teams to embrace data-driven maintenance practices.
Challenges and Considerations
While AI-driven predictive maintenance offers numerous benefits, it also presents several challenges:
- Data Quality:The accuracy of AI predictions depends on the quality of data. Ensuring that data is clean, accurate, and consistent is crucial for effective predictive maintenance.
- Integration with Legacy Systems:Many manufacturing facilities operate with legacy equipment and systems that may not be compatible with modern AI technologies. Integrating AI with these systems can be complex and require significant investment.
- Initial Costs:Implementing AI-driven PdM involves upfront costs for sensors, data infrastructure, and algorithm development. Manufacturers must assess the long-term ROI to justify these investments.
- Skill Requirements:Developing and maintaining AI-driven PdM systems require specialized skills in data science, machine learning, and maintenance engineering. Addressing the skills gap through training and hiring is essential.
Future Trends and Opportunities
The future of AI-driven predictive maintenance looks promising, with ongoing advancements expected to further enhance its capabilities:
- Edge Computing:Edge computing allows data processing to occur closer to the source of data generation, reducing latency and enabling faster decision-making. This is particularly useful for real-time predictive maintenance applications.
- Integration with Digital Twins:Digital twins are virtual replicas of physical assets that simulate their behavior in real-time. Integrating AI-driven PdM with digital twins can provide deeper insights into equipment performance and optimize maintenance strategies.
- AI-Powered Autonomous Maintenance:The evolution of AI could lead to autonomous maintenance systems where AI not only predicts but also performs maintenance tasks with minimal human intervention. This could revolutionize maintenance practices and further reduce downtime and costs.
Conclusion
AI-driven predictive maintenance is transforming the manufacturing industry by enabling proactive, data-driven maintenance strategies that enhance efficiency, reduce costs, and improve safety. By leveraging advanced analytics and real-time monitoring, manufacturers can predict and prevent equipment failures, ensuring optimal performance and longevity of machinery. While the implementation of AI-driven PdM presents challenges, the benefits far outweigh the obstacles, making it a worthwhile investment for forward-thinking manufacturers. As AI technology continues to evolve, its role in predictive maintenance will only grow, offering new possibilities for innovation and excellence in manufacturing operations.